Predictive analytics is the application of statistical techniques, machine learning algorithms, and data mining to analyze historical data and make predictions about future events. In sports, predictive analytics uses data-driven insights to anticipate athlete performance, team dynamics, and injury risks. As sports become more data-driven, harnessing predictive analytics is essential for teams and organizations. It helps maximize performance potential.
Predictive analytics in sports is based on the idea that past performance can predict future success. By analyzing historical data, athletes and coaches can identify trends, strengths, and weaknesses. This allows them to create customized training programs aligned with performance goals. In today’s competitive environment, using predictive analytics is essential for achieving excellence.
Understanding Sports Performance Tracking
Sports performance tracking involves the systematic collection and analysis of data related to an athlete’s performance during training and competition. This process captures a wide range of metrics, including physical, physiological, and biomechanical data. Modern performance tracking uses technologies like wearable devices, sensors, and video analysis to gather precise data. Wearables, such as GPS trackers and heart rate monitors, are key to this process. These devices provide real-time insights into an athlete’s movements, exertion, and physiological responses. Coaches and analysts can use this data to monitor performance with high accuracy. It helps identify trends, adjust training, and ensure athletes are ready for competition.
How Predictive Analytics Enhances Sports Performance
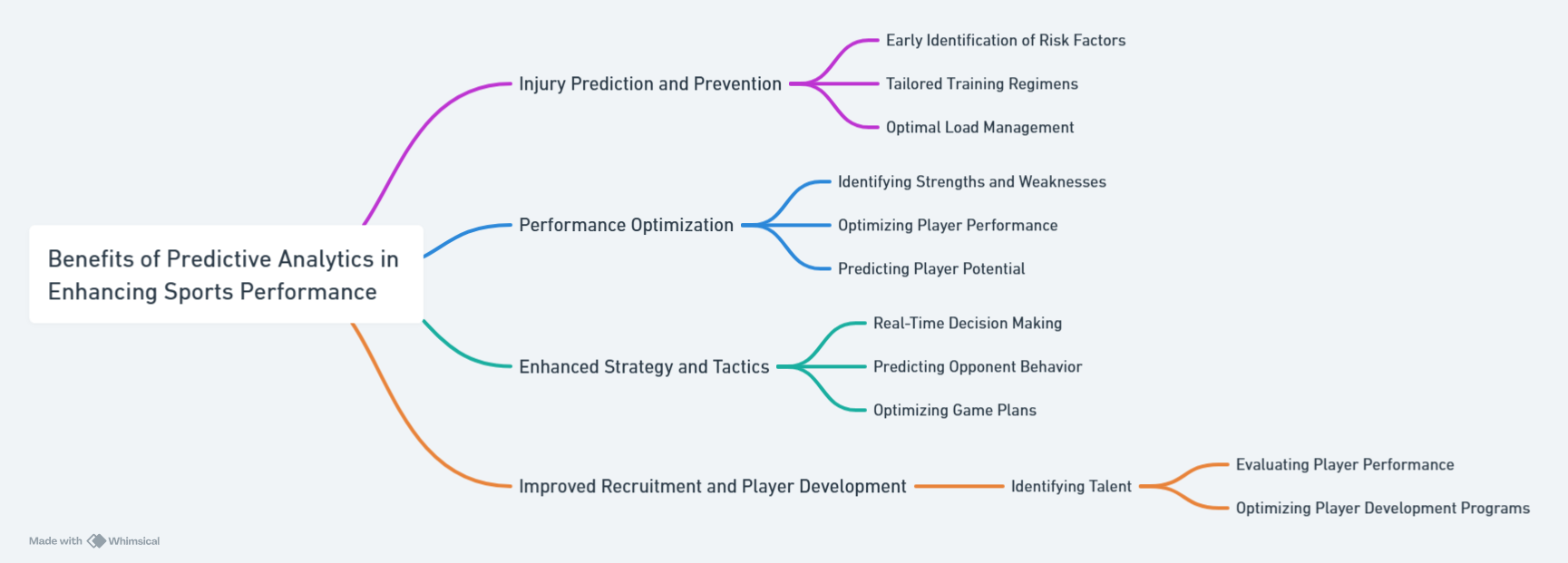
Predictive analytics enhances sports performance by transforming raw data into actionable insights. By employing statistical models and machine learning algorithms, predictive analytics can identify patterns and correlations within performance data, enabling athletes and coaches to make informed decisions. The global sports analytics market size was valued at USD 3.78 billion in 2023 and is projected to reach from USD 5.64 billion in 2024 to USD 32.31 billion by 2032, registering a CAGR of 26.92% during the forecast period (2024-2032). Here are some key ways predictive analytics contributes to enhancing sports performance:
Tailored Training Programs
Through the analysis of historical performance data, coaches can identify individual athletes’ strengths and weaknesses, leading to the development of personalized training programs. Predictive analytics allows for the optimization of training loads, ensuring athletes are neither overworked nor under-challenged. By predicting how different training regimens will impact performance, coaches can fine-tune training plans to maximize gains and minimize the risk of injury.
Injury Prevention
One of the most significant benefits of predictive analytics in sports is its application in injury prevention. By analyzing data related to physical exertion, fatigue levels, and biomechanics, predictive models can identify early warning signs of potential injuries. For example, if an athlete’s workload surpasses a set threshold, predictive analytics can alert coaches. This prompts them to adjust training intensity or add recovery time. This proactive approach helps reduce injuries, extending athletes’ careers and improving team performance.
Game Strategy and Decision-Making
Predictive analytics plays a vital role in shaping game strategies and in-game decision-making. Coaches can analyze opponents’ historical performance data to identify patterns, strengths, and weaknesses. By leveraging predictive models, coaches can develop game plans that exploit opponents’ vulnerabilities while capitalizing on their team’s strengths. Additionally, real-time data analysis during games allows coaches to make immediate adjustments based on performance metrics, leading to more informed tactical decisions.
Performance Monitoring
Continuous performance monitoring is crucial for athletes’ success. Predictive analytics enables coaches to track athletes’ progress over time, assessing how training changes impact performance metrics. By identifying trends in performance data, coaches can gauge whether athletes are meeting their goals or if adjustments are necessary. This ongoing evaluation fosters a culture of accountability and improvement, encouraging athletes to take ownership of their performance.
Role of Data Science in Sports Analytics
Data science is at the heart of predictive analytics in sports. It encompasses the collection, processing, and analysis of vast amounts of data to derive meaningful insights. Data scientists work closely with coaches and sports analysts to develop predictive models that reflect each athlete’s unique needs and team dynamics.Machine learning algorithms are instrumental in data science for sports analytics. These algorithms can learn from historical data, adapt to new patterns, and improve their predictive accuracy over time. By employing techniques such as regression analysis, clustering, and classification, data scientists can uncover hidden insights within complex datasets, driving better decision-making processes.
Types of Data Used in Sports Performance Tracking
Sports performance tracking involves various types of data collection, each contributing valuable insights into an athlete’s performance. The following are the primary data types utilized in predictive analytics:
Physical Metrics
Physical metrics include speed, acceleration, distance covered, and movement patterns. These measurements provide insights into an athlete’s physical capabilities and can be tracked using wearable devices such as GPS units and accelerometers.
Physiological Data
Physiological data encompasses heart rate, blood pressure, and lactate levels, all of which offer insights into an athlete’s cardiovascular health and overall fitness levels. Monitoring these metrics allows coaches to assess training intensity and recovery needs.
Biomechanical Data
Biomechanical data focuses on an athlete’s movement mechanics, including joint angles, muscle activation patterns, and posture. Analyzing biomechanical data helps identify areas for improvement, enabling targeted interventions to enhance performance and reduce injury risks.
Gameplay Data
Gameplay data refers to statistics derived from in-game performance, such as possession time, shot accuracy, and pass completion rates. Analyzing gameplay data allows teams to assess performance relative to game strategies and develop tactical adjustments.
Psychological Metrics
Psychological metrics, including mood, stress levels, and sleep quality, provide insights into an athlete’s mental state. Mental preparedness is crucial for peak performance, and tracking these metrics can help coaches support athletes’ overall well-being.
Key Metrics in Predictive Sports Analytics
Several key metrics play a critical role in predictive sports analytics. Focusing on these metrics allows coaches to tailor training programs and game strategies to optimize performance:Velocity and AccelerationHigh-frequency tracking of velocity and acceleration enables coaches to assess athletes’ speed and agility, making it easier to optimize positioning and workload during training and competition.Endurance and StaminaMetrics related to endurance, such as heart rate variability and oxygen consumption rates, provide insights into an athlete’s ability to sustain effort over time. Understanding these metrics helps coaches develop training programs that improve stamina.AgilityAgility metrics track an athlete’s reaction times and directional changes, which are essential in sports requiring quick movements. These metrics can inform training strategies to enhance overall agility.Injury Prediction MetricsPredictive models can leverage fatigue levels, workload, and joint stress metrics to identify athletes at risk of injury. Monitoring these metrics proactively can help mitigate injury risks and prolong athletic careers.
Wearable Technology in Data Collection
Wearable technology has transformed sports performance tracking, allowing athletes to collect real-time data directly from their bodies. Devices such as GPS trackers, smartwatches, and heart rate monitors provide continuous insights into an athlete’s performance metrics. The accessibility and accuracy of wearables enable teams to monitor athletes’ conditions closely, informing data-driven decisions that enhance performance.Wearable devices can track various metrics, including speed, heart rate, and movement patterns, giving coaches a comprehensive view of an athlete’s performance. By integrating this data into predictive analytics models, coaches can gain deeper insights into individual athlete profiles and overall team performance.
AI and Machine Learning in Sports Performance
Artificial intelligence (AI) and machine learning are revolutionizing the field of sports analytics. These technologies enable the processing of vast amounts of data and the identification of complex patterns that might go unnoticed by traditional analysis methods. Machine learning algorithms can learn from historical performance data and adapt to new trends, improving their predictive accuracy over time.AI can also enhance real-time decision-making during games. By analyzing player movements and game conditions, AI-driven systems can recommend tactical adjustments based on real-time performance metrics. This level of responsiveness gives teams a strategic edge, allowing them to adapt quickly to evolving game situations.
Predictive Analytics for Injury Prevention
Injury prevention is a paramount concern in sports, and predictive analytics plays a vital role in reducing injury risks. By analyzing physical and physiological data, predictive models can identify early warning signs of potential injuries. For example, if an athlete’s workload exceeds a certain threshold, predictive analytics can alert coaches. This prompts them to adjust training intensity or provide extra recovery time. Additionally, predictive analytics can analyze patterns of previous injuries. This helps teams develop personalized preventative strategies. By taking a proactive approach to injury management, teams can reduce injury risk, improve athlete longevity, and enhance overall performance.
Tracking Performance Across Different Sports
The application of predictive analytics varies significantly across different sports, as each sport has unique demands and performance indicators. For example, in sports like basketball and football, speed and agility are critical, while endurance and stamina are paramount in long-distance running. Tailoring predictive analytics to the specific requirements of each sport ensures the relevance and accuracy of insights, allowing athletes to optimize performance within their specific contexts.
Example: Football vs. Basketball
In football, predictive analytics focuses heavily on analyzing positional play, defensive and offensive strategies, and the physical demands of the game. Metrics like player positioning, speed, and tackling efficiency are key for developing game strategies. In basketball, analytics focus on shooting accuracy, offensive efficiency, and defensive matchups. Predictive models analyze shot selection, player movement, and team dynamics. This enhances strategic decision-making during games.
Importance of Real-Time Data in Decision-Making
Real-time data is a game-changer in sports performance tracking, allowing coaches and athletes to make informed decisions on the fly. The ability to monitor performance metrics during training and competition enables immediate adjustments to training regimens, game strategies, and athlete management. For example, if an athlete’s heart rate spikes during a game, coaches can make tactical adjustments to alleviate fatigue or injury risks.Additionally, real-time data facilitates more dynamic coaching approaches, fostering better communication between coaches and athletes. Coaches can provide instant feedback based on performance metrics, allowing athletes to adjust their strategies and tactics in real time.
Conclusion
Predictive analytics is transforming sports performance tracking, enabling athletes and coaches to harness data-driven insights for improved performance and strategic decision-making. By integrating various data types and employing advanced analytical techniques, sports organizations can enhance training programs, prevent injuries, and optimize game strategies. As technology continues to evolve, the potential for predictive analytics in sports is limitless, paving the way for a new era of data-driven athletic excellence.Through its ongoing integration into sports, predictive analytics is not just a tool but a critical component of achieving peak performance and fostering athlete development in a highly competitive landscape. Embracing this data-driven approach will ultimately empower athletes, coaches, and teams to reach new heights in their pursuit of excellence.
FAQs
How can predictive analytics improve performance?
Predictive analytics improves performance by using historical data and statistical algorithms to forecast future outcomes. This allows organizations to make informed decisions, optimize operations, and anticipate needs, ultimately enhancing efficiency and reducing costs. In fields like sports, healthcare, and business, predictive analytics can identify trends, improve strategies, and allocate resources effectively to boost performance.
What are examples of prescriptive analytics in sports?
Prescriptive analytics in sports uses data to recommend actions that improve team strategy and player performance. Examples include optimizing player rotation based on fatigue levels to reduce injury risk, suggesting lineup adjustments to counter opponents’ weaknesses, and developing game plans that maximize win probabilities. Prescriptive analytics transforms data into actionable strategies, helping teams and players gain a competitive edge.
How can data analytics be used in sports?
Data analytics in sports helps improve team performance, fan engagement, and player health. Teams analyze player stats and game footage to refine tactics and optimize training. Data is also used for injury prevention by monitoring physical indicators. Additionally, analytics enhance fan experience through personalized content and ticketing, improving overall engagement and loyalty.
What is the most used technique in predictive analytics?
Regression analysis is one of the most widely used techniques in predictive analytics. It establishes relationships between variables, allowing analysts to predict outcomes based on influencing factors. Other common techniques include time series analysis for trend forecasting and machine learning algorithms, which improve prediction accuracy by learning from large datasets.